Welcome back to our AI and Diversity & Inclusion collection, a three part blog series that dives into the relationship between Artificial Intelligence and Diversity and Inclusion topics. This week we are discussing: Bias.
Similar to last week, Diversein has also collaborated with two ambassadors from Women in AI, Begüm and Nabanita, to provide an inside perspective. Click here to find out more about Women in AI!
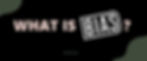
According to the Cambridge dictionary, bias is "the action of supporting or opposing a particular person or thing in an unfair way, because of allowing personal opinions to influence your judgment." Bias can manifests in two main forms: conscious and unconscious bias.
Conscious bias is a type of bias that usually comes with an awareness to preferences or prejudices, meaning they are 'conscious' thoughts and beliefs we hold and express through our actions. An example of this is a startup not hiring people over a certain age because they believe only certain age groups are 'innovative'.
Unconscious bias is thus bias that we develop through how we were raised, our environment, experiences, etc that shapes our beliefs in an automatic way, and we may not even be aware that we have these biases. An example of this the 'Halo Effect', which is when we attribute characteristics to someone based on how they look. When we see someone with glasses, we unconsciously assume they are smart, if we see someone smiling, we assume they are friendly, etc.
There is also bias in various contexts, such as gender or sexual orientation related bias, racial bias, and so forth. However, bias is not necessarily a bad thing. It's important to note that all human beings naturally have biases, some of which we can identify and challenge, and some that are reproduced without awareness.
Functionally, biases are established patterns of identifying or codifying the world that our brains do automatically, in order to make quick decisions and understand our surroundings.
We should always be careful to challenge our biases and be mindful about their origins, their validity, etc. When our biases are presented as fact without challenging any potentially harmful notions, they can include harmful stereotypes or misconceptions.
The issue with reproducing these biases is that AI then learns from this input during data collection, and can perpetuate these harmful ideas.
Question: In your opinion, what are the possible biases that may emerge within AI concerning diversity and inclusivity?
Begüm: Possible biases that emerge within AI regarding diversity and inclusion are rooted in historical societal biases inherent in the data AI systems consume. AI often requires labeled data, and these labels are introduced by humans, who can bring their biases. Consequently, AI's future predictions or analyses might reflect these biases. A prime example is Timnit Gebru's groundbreaking work on "gender shades," revealing that if our data isn't inclusive, AI systems can exhibit differential behavior for different users. Ensuring diversity in training data is critical to mitigate these biases. AI should be designed to recognize and address underrepresented groups, avoiding perpetuation of inequalities. Proper auditing and testing of AI systems for fairness and inclusivity are essential. This becomes especially significant when AI is used in assessments, hiring, or criminal justice, as these decisions can have far-reaching implications.
Nabanita: In AI, if we talk about the industrial applications that I have worked on like fintech and healthcare, that element is always there. If you're processing transaction data, user profiles, health indicators and everything associated from that standpoint, it's extremely critical to remove all those biases. There's a whole school of a wider discussion, but I think we have taken very conscious steps towards this transformation in recent times and people are thinking about it and taking it seriously.
They want feedback about it and now we've got a lot of feedback chains in big data. The way we speak and the way we think as humans is always biased and that flows through the data that we have as well, because there's huge natural language data flowing from people like you and me and, of course, none of us are free from biases. So that feeds on when you train any kind of AI. When AI seems unbiased, it indicates that it's not random but has been well-engineered. So we're definitely heading towards a better system and a society.

Artificial Intelligence has shown the potential to diminish the human element of subjectively interpreting data and promote fairness, provided they are trained with well designed algorithms. Unlike humans, machine learning systems do not rely on emotions, personal history or hidden agendas when making choices, and instead focuses solely on predicting outcomes based on the available data. If the right metrics of fairness are provided, AI should be able to make a logical, equitable decision that a human may not be able to when faced with a problem.
The complexity also lies here, as a complete set of terms that define 'fairness' does not exist. Rather than pushing for a blanket metric to measure fairness, it is instead imperative to adjust methods based on a "holistic approach", and train AI with a variety of vetted sources in order to maintain balance. Google states that a holistic approach for AI and fairness includes:"...fostering an inclusive workforce that embodies critical and diverse knowledge, to seeking input from communities early in the research and development process to develop an understanding of societal contexts, to assessing training datasets for potential sources of unfair bias, to training models to remove or correct problematic biases, to evaluating models for disparities in performance, to continued adversarial testing of final AI systems for unfair outcomes."
Question: Are you aware of any measures to monitor and evaluate the performance of AI systems for potential disparities across various demographic groups?
Begüm: Monitoring and evaluating AI systems for potential disparities across demographic groups is crucial for ensuring fairness and equity. In today's AI landscape, data quality is paramount. EU-funded projects, for instance, increasingly prioritize robust data quality plans as a foundation. While ongoing research focuses on data quality assurance, actionable approaches are emerging. To assess disparities, proactive steps include engaging underrepresented communities during and after AI development, performing statistical and sensitivity analyses, and meticulously examining data for any inherent biases. By embracing representative datasets, measuring prediction disparities, and striving for fairness, AI systems can progress toward mitigating potential disparities. Incorporating user feedback, implementing updates, and providing transparent explanations are integral to fostering responsible and unbiased AI solutions.

As AI is trained on content produced by humans, there is a chance for societal or historical inequities to be absorbed via machine learning.
For example, a University of Washington study found that, when sourcing image data from Google, the top search results for 'CEO' were predominantly male, with only 11% of the images including women. However, currently 27% of U.S. CEOs are women, leading to a gender disparity in female representation. The prevalence of female or male representation based on keyword searches tended to vary, however the 'CEO' search image case has the potential to reinforce stereotypes and societal perceptions on women's roles.
The quality of data and the diversity of it heavily contributes as well to the outputs of AI, as without it algorithmic discrimination can run rampant. In this facial analysis study, it was found that the facial recognition datasets the IBM classifier AI was trained on was overwhelmingly composed of lighter-skinned individuals, leading to higher error rates when identifying minorities, particularly women of color, with error rates of up to 34.7%.
Question: How can we ensure AI-generated content respects cultural sensitivities and avoids stereotypes?
Nabanita: I think similar research is being conducted right now, for example on social media. Social media is one of the biggest areas where things can go massively wrong in uncontrolled environments. In most controlled environments we would not deal with situations like that. But when it comes to social media, there's no control over what people post and how people share their opinions in public. I know that there are a few applications like hate speech detection, for example. Instagram automatically classifies certain videos as inappropriate based on content, violence or graphic images they believe might cause mental trauma, and it automatically gives a disclaimer on it and that works on the feedback from crowd reporting.
After X number of reports, they flag the video and take note of the feedback. Crowd-sourcing is a great way to tackle these issues and I see many people actually reviewing and flagging content on the Internet and contributing to the greater good for society.

So what does this mean going forward? Well, it's evident that the relationship between AI and bias is a complex yet critical point we address as we begin to use it more and more for everyday, automated tasks. There is a strong potential for Artificial Intelligence to make great strides in reducing human bias and promoting equity, but we have to tread carefully and be selective with the kind of data we use, vetting it so that our AI systems do not inherit any discriminatory practices. Just as AI could have a positive impact on our society, the potential consequences for misuse are just as, if not greater.
Having recently finished an internship in Human Resources, Amanda Mola is a Diversity and Inclusion Resource Manager at Diversein, creating content about the D&I industry and fostering inclusive intelligence. You can connect with her here: https://www.linkedin.com/in/amanda-mola-977282155/